Problem Modeling to inform research design
Very early on in my career, while working at BlackBerry, I received a nugget of wisdom that fundamentally changed how I thought about the practice of design:
"you are very good at solving problems... but you need to find the right problems to solve."
This statement hit me like a proverbial brick. It fundamentally shifted my conception of design as a field, away from purely aesthetic sensibilities and towards an approach to systematically thinking through problems. It also taught me to be careful about wasting time-solving the wrong problems, not to mention sparking a fascination with thinking about how people think.
Problem Modeling and Research
1/17/23
"All models are wrong, but some are useful"
- George Box.
Most problems – particularly those in the design or innovation space – aren't typically one-dimensional. They're multifaceted, complex, ambiguous, and weird, laden with interconnected (often hidden) variables; shaped by unknown factors or forces. Thinking through any kind of complexity requires a particular mindset - one that is focused on first principles, root causes, and relationships. I refer to this form of systems thinking as Problem Modeling.
We use mental models daily to help us think through the complexity of the world. They serve as internal cognitive sketches, a sort of mental short-form to synthesize what we know about a given phenomenon into a rough working model that provides predictive ability or helps us make decisions.
Problem Models are conceptual tools, representations that we build in our mind to guide us in what we know, assume, or don't know about a given problem. This includes its effects, causes, nuance, and mitigating factors that amplify or diminish it. It's "what's going on under the surface" of a problem, so to speak.
The better we can model or understand a problem (and its intricate nuance), the more accurately we can diagnose its inner workings and propose successful solutions. Unfortunately, we don't often spend much time thinking and building good problem models as a habit, even though we should. Off the cuff, unconsidered assessments of a problem or situation can have pretty adverse effects.
Imagine a time-poor doctor who makes an incorrect diagnosis for a patient. Whatever prescription or treatment they administer isn't likely to lead to an effective cure or – even worse – produce unnecessary and potentially dangerous side effects (difficulty modelling biological systems is part of why medicine is so tough). It would be literally solving the wrong problem.
Building good models allows us to discern and explore the multifaceted layers and interconnected dynamics of a problem, ensuring we don't default to oversimplified solutions for complex challenges. Conversely, poorly understood problem models are unlikely to lead to effective design solutions. In fact, an incomplete or misguided understanding of the dynamics of a problem can lead to interventions that actually make the problem much worse (not to mention waste time and money).
So what?
It's my belief that the education system (K12 to Post Secondary) doesn't really prioritize modelling problems, only solving them. This missing cognitive skill or habit leads to a lot of wasteful and dumb work in our jobs and everyday lives and drives deeper, more subtle issues with society as a whole (but that's a post for another day).
I would go as far as to say that modelling problems before we start to solve them are a key skill required by all modern design, research, and innovation teams (especially in agile environments). Even a clear definition of what we are trying to solve can do wonders in aligning teams and ensuring more successful outcomes.
This is why I've been working to form "problem modelling" into a process/framework that can inform design/research work and general knowledge workers across the board.
Applying this thinking can be as simple as a few questions at the start of any project: who is involved; what is their context, situation, or problem; what do they want; and (based on all of that) what should we seek to learn, validate, or better understand to help them?
Of course, the answers to each of these questions are almost always assumptions to a varying degree, but that's not the point. They help provide us with a rough set of first principles from which we can determine our research inquiry (and eventually recommend interventions). In a sense, these foundational questions represent a first rough sketch of our initial conceptual model of whatever is going on (for the client) — the problem, context, or situation that needs to be resolved.

I find it helpful to imagine this "model" as a nebulous blob or cloud in floating space. It may have areas that are dense and tightly resolved with detail. These represent what we "know" or are at least more confident about regarding the problem, context, or situation. It also contains areas of blurriness, sparsity, or lower density. These represent areas where we have things we think are true or feel right, but we don't know for sure — they're our assumptions.
Crucially, our nebulous cloud also has areas where there is nothing but emptiness. These represent aspects of the problem, context, or situation where we have no idea, data, or realistic assumptions to go on. These are things we know that we don't know or unknown things we don't even know exist but need to consider to avoid nasty surprises.
I like to think of this higher-dimensional nebula abstraction as a kind of holographic representation or "shape" of the problem. Each client problem, context, or situation has its own shape, a unique distribution of density (knowns), sparsity (assumptions), and empty areas (unknowns). This shape gives us a nearly perfect list of what research activities to perform.
And that's the point. By modelling the problem, we can systematically determine how complete (or incomplete) our understanding is. In my mind, all research activities are just ways of gathering information that fills in or completes our problem model or, put another way: to better focus and define our nebulous problem "shape." Once we have this, we can start setting up the right research with the right people, learning and filling our gaps in knowledge.
No wasted time, gut instinct, or guesswork, just building our understanding where we need it most. As a bonus, solutions and suitable interventions become pretty obvious as our knowledge of the problem improves, and the risk of focusing on the wrong things is significantly reduced.
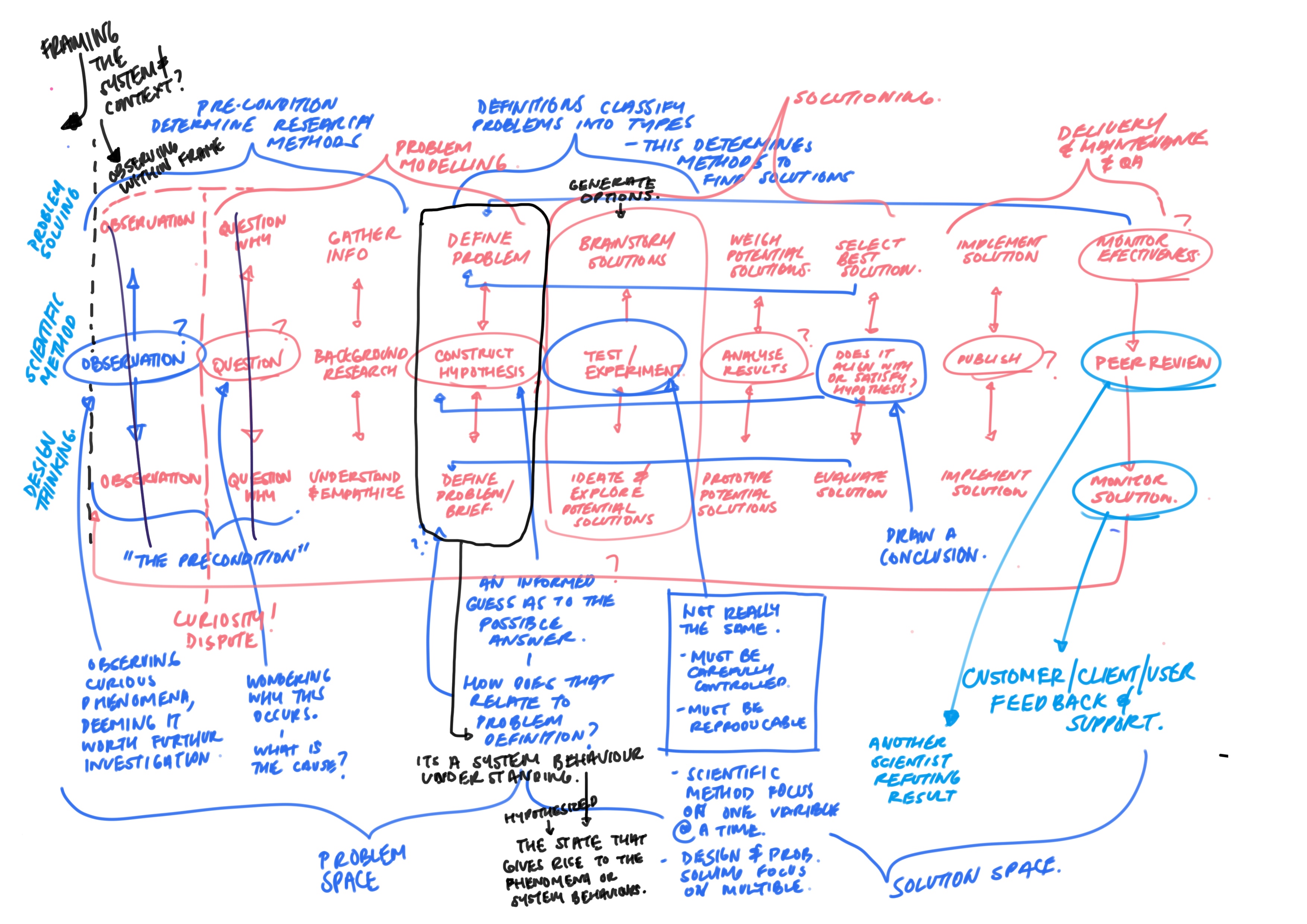
So how can you ensure that you are solving the right problems? Begin by asking the following question at the beginning of your next project:
- Who is involved?
- What is their context, situation, or problem?
- What do they want?
Make sure to think critically about the answers - that will help you classify them as knowns, assumptions, or unknowns:
- Are any of the answers to these questions actually true?
- How do we know? And what data do we have to support that?
- If not, how do we find out or gather data to validate that?
- What else might we need to learn about?
- And so on…
Problem modelling is a critical tool for understanding and addressing complex issues. It helps us avoid oversimplification, guides us toward more accurate and practical solutions, and safeguards against the risks of rushed, poorly informed decisions. It requires time and thought, but its value in navigating complexity and fostering innovation makes it an indispensable tool in design, technology, and strategic innovation.